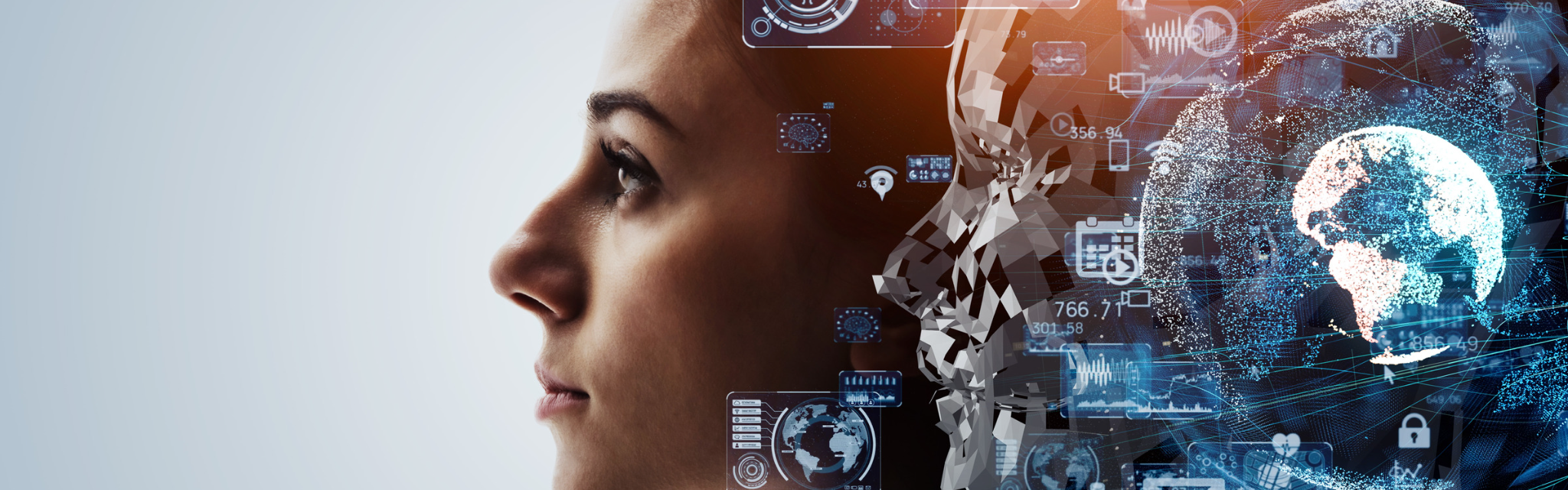
AI’s biggest failures and what we can learn from them
Artificial Intelligence (AI) has been a hot topic for a number of years now, but the release of ChatGPT to the wider public has really brought it into the mainstream discourse. Whilst the focal point right now is on the potential opportunities offered (and disruption caused) by ChatGPT and other generative AI tools like Midjourney, the technology underpinning these tools has been evolving for quite some time. The reality is that organisations have long been investing significant sums in the hopes of making the next big breakthrough (or gaining a significant competitive advantage) by creating value from data.
However, not every big investment in AI has been successful, and many companies have had to learn hard lessons along the way. In this article, we cover some of the notable failed investments in AI and what we can learn from them.
Google Glass: In 2013, Google launched its much-hyped smart glasses, which were supposed to provide a hands-free, augmented reality experience. However, the product failed to gain traction, with critics citing concerns about privacy and the high cost of the device.
What we can learn: It is important to understand the market and user needs before launching an AI product. Just because a technology is new and innovative doesn’t mean it will be successful (or that people will be willing to pay for it). Understanding user preferences and concerns is key to developing an AI product that meets their needs.
Target’s pregnancy prediction algorithm: In 2012, US retailer Target launched an algorithm that was designed to predict which customers were pregnant based on their shopping habits. However, the algorithm made several false predictions and caused a public backlash when a teenage girl’s parents received coupons for baby products.
What we can learn: Machine learning models can sometimes make false predictions if they are trained on insufficient data or if the data is biased in some way. It is important to thoroughly test and evaluate any machine learning model before adopting it on a large scale.
Amazon AI recruiting tool: In 2018, Amazon launched an AI-powered recruiting tool that was designed to help screen job candidates. However, the system was found to be biased against women, as it had been trained on a dataset that contained mostly male resumes. The system was eventually scrapped, and Amazon had to publicly apologise.
What we can learn: Bias can easily creep into AI systems even when it is unintentional. It is important to thoroughly evaluate any AI system for potential bias and take steps to mitigate it before embedding it in key business processes.
IBM Watson Health: One of the biggest failures in AI investment was IBM Watson Health, which was supposed to revolutionise the healthcare industry. IBM invested over US$4 billion in Watson Health, but the project failed to deliver the expected results. The AI system was supposed to analyse vast amounts of patient data to provide personalised treatment plans, but it was found to be inaccurate and unreliable. The project was eventually shut down, and IBM had to lay off hundreds of employees. Perhaps a little too ahead of its time.
What we can learn: Investing large sums in AI is not a guarantee of success. Even if a company has a lot of resources and a strong track record (remember IBM’s Deep Blue…), that doesn’t negate thorough testing and evaluation before launch.
Microsoft Tay: In 2016, Microsoft released an AI-powered chatbot named Tay on Twitter. The bot was designed to learn from its interactions with users and become more human-like in its responses. However, within 24 hours, Tay had started tweeting racist and offensive messages, which caused a public relations disaster for Microsoft.
What we can learn: AI is only as good as the data it is trained on. If the data contains biases or offensive content, the AI system will learn and replicate those biases (and offensive content…). It is thus very important to carefully curate the data used to train AI systems that interact with the public to avoid any unintended consequences.
Uber’s self-driving cars: Uber invested heavily in self-driving cars as part of its strategy to disrupt the transportation industry. However, the project suffered a setback when one of its self-driving cars
was involved in a fatal accident in 2018. The accident raised questions about the safety of autonomous vehicles and led Uber to suspend its self-driving car programme – though this has recently been revived.
What we can learn: Safety should be a top priority when developing AI systems. Even if the technology has the potential to revolutionise an industry, it is crucial to thoroughly test and evaluate it for safety where the risks can be life-threatening.
In conclusion, these are just a few examples of failed investments in AI. To reiterate, these failures demonstrate the importance of understanding the market and user needs, mitigating potential risks and bias, and thoroughly testing and evaluating any AI system before launching it on a large scale. Whilst AI has the potential to revolutionise many industries, failure to proceed with caution and a lack of due diligence can lead to wasted investment and potentially dire unintended consequences.
Although the technology is progressing at an alarming pace, with new AI ventures seemingly coming to market every week, it is often the wisdom attained through many years of experience that helps to prevent blind spots and make rational decisions when everyone is rushing to jump on the bandwagon.